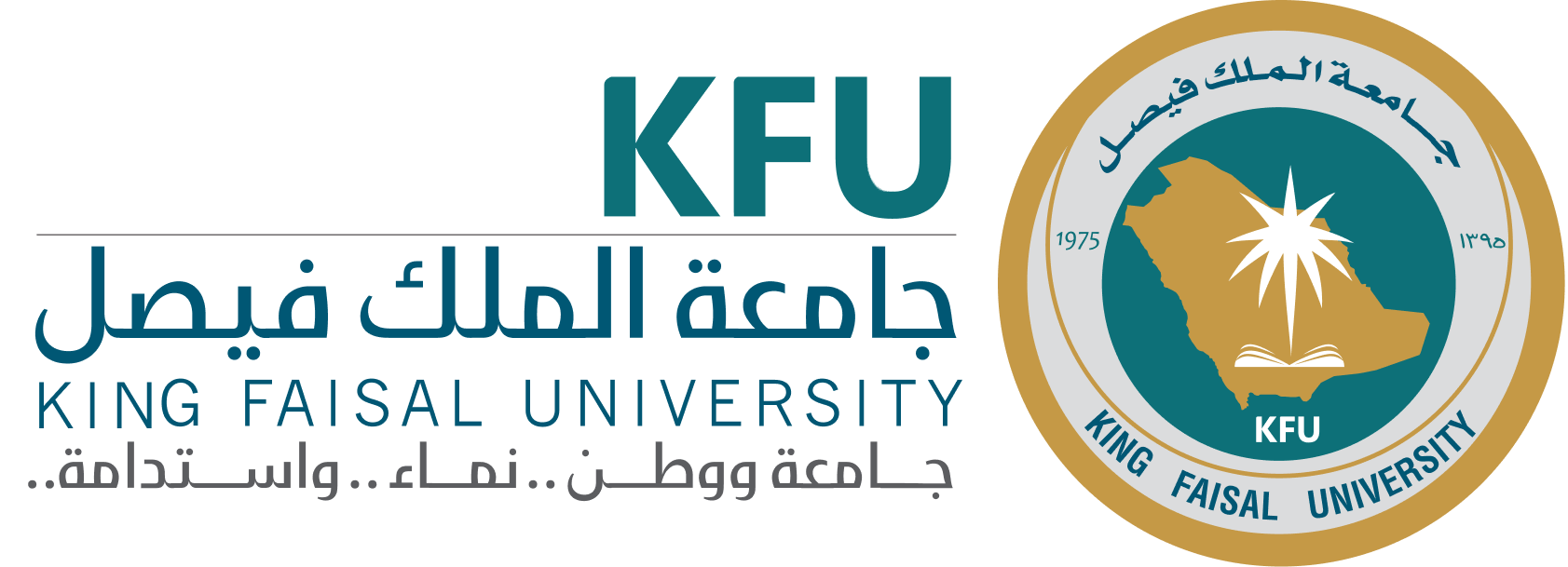
Scientific Journal Of King Faisal University: Basic and Applied Sciences
Scientific Journal of King Faisal University: Humanities and Management Sciences
Methods of Overcoming Data Waste in Education: A Systematic Review
(Aminah S. Aldossary and Leena A. Alfarani)Abstract
This research aimed to perform a systematic review of recent scientific studies that addressed the topic of educational data mining by highlighting recent research trends and reviewing the best practices of smart technology in this field. The current research was restricted to scientific studies and conferences in English that were published through the IEEE database between 2020 and October 2022. After applying the PRISMA form and reviewing and documenting the method, 25 papers were identified that matched the criteria. The outcomes of the current research concluded that the trend of prediction was one of the most common research trends in the domain of educational data mining. Moreover, this trend varied remarkably in its coverage of educational scientific aspects. On the other hand, the outcomes demonstrated that the trend of recommending the most appropriate specialised tracks for learners was one of the least common research trends. With regard to intelligent technical practices, the outcomes of the research revealed that the most used and mature intelligent technologies in practical frameworks and algorithms were intended for prediction purposes, and these were shown to greatly enhance researchers’ ability to reach accurate and logical results that are applicable in real educational contexts.
KEYWORDS
Artificial intelligence, machine learning, algorithms, neural networks, educational data, educational institutions
PDF
References
Al Ulwan, J.A. (2020). Aliatijahat al'iidariat almueasirat fi tanmiat almawarid albashariat murajaeatan manhajiatan lil'adabiaat dhat alealaqa 'Contemporary administrative trends in the development of human resources a systematic review of the relevant literature'. Journal of Humanities and Social Sciences, n/a(46), 119–67. [in Arabic]
Lal, Z.Y. and Aljindi, A.A. (2005). Alittesal Alelictroni w Tiknolojya Altaleem ‘Electronic Communication and Education Technology’. Riyadh, Saudi Arabia: Obeikan Bookstore. [in Arabic]
Wathīqah Altaḥawwul Alrqmy. (2020). Roayat Almmlkt 2030 ‘Kingdom Vision 2030’. Available at: https://vro.moenergy.gov.sa/Arabic/DocLib/NTP_ar.pdf (accessed on 23/ 11 /2022) [in Arabic]
Abdelkader, H., Gad, A., Abohany, A. and Sorour, S. (2022). An efficient data mining technique for assessing satisfaction level with online learning for higher education Students during the COVID-19. IEEE Access, 10(n/a), 6286–303.
Araujo, L., Lopez-Ostenero, F., Martinez-Romo, J. and Plaza, L. (2020). Deep-learning approach to educational text mining and application to the analysis of topics difficulty. IEEE Access, 8(n/a), 218002–14.
Buenano-Fernandez, D., Gonzalez, M., Gil, D. and Lujan-Mora, S. (2020). Text mining of open-ended questions in self-assessment of university teachers: An LDA topic modeling approach. IEEE Access, 8(n/a), 35318–30.
Chen, H., Nguyen, B., Yan, Y. and Dow, C. (2020). Analysis of learning behavior in an automated programming assessment environment: A code quality perspective. IEEE Access, 8(n/a), 167341–54.
Czibula, G., Ciubotariu, G., Maier, M. and Lisei, H. (2022). IntelliDaM: A machine learning-based framework for enhancing the performance of decision-making processes. A case study for educational data mining. IEEE Access, 8(n/a), 80651–66.
Du, X., Yang, J. and Hung, J. (2020a). An integrated framework based on latent variational autoencoder for providing early warning of at-risk students. IEEE Access, 8(n/a), 10110–22.
Du, X., Yang, J., Hung, J. L. and Shelton, B. (2020). Educational data mining: A systematic review of research and emerging trends. Information Discovery and Delivery, 48(4), 225–36.
Feng, G., Fan, M. and Ao, C. (2022). Exploration and visualization of learning behavior patterns from the perspective of educational process mining. IEEE Access, 10(n/a), 65271–83.
Ghorbani, R. and Ghousi, R. (2020). Comparing different resampling methods in predicting students’ performance using machine learning techniques. IEEE Access, 8(n/a), 67899–911.
Gronberg, N., Knutas, A., Hynninen, T. and Hujala, M. (2021). Palaute: An online text mining tool for analyzing written student course feedback. IEEE Access, 9(n/a), 134518–29.
Iqbal, N., Jamil, F., Ahmad, S. and Kim, D. (2020). Toward effective planning and management using predictive analytics based on rental book data of academic libraries. IEEE Access, 8(n/a), 81978–96.
Kumar, R. and Sharma, A. (2017). Data mining in education: a review. International Journal of Mechanical Engineering and Information Technology, 5(1), 1843–5.
Li, Z., Li, Y. and Xie, Z. (2020). Exploring the significant predictors to the quality of master’s dissertations. IEEE Access, 8(n/a), 21152–8.
Mengash, H. (2020). Using data mining techniques to predict student performance to support decision making in university admission systems. IEEE Access, 8(n/a), 55462–70.
Nabil, A., Seyam, M. and Abou-Elfetouh, A. (2021). Prediction of students’ academic performance based on courses’ grades using deep neural networks. IEEE Access, 9(n/a), 140731–46.
Prabowo, H., Hidayat, A., Cenggoro, T., Rahutomo, R., Purwandari, K. and Pardamean, B. (2021). Aggregating time series and tabular data in deep learning model for university students’ GPA prediction. IEEE Access, 9(n/a), 87370–7.
Prada, M., Dominguez, M., Vicario, J., Alves, P., Barbu, M. and Podpora, M. (2020). Educational data mining for tutoring support in higher education: A web-based tool case study in engineering degrees. IEEE Access, 8(n/a), 212818–36.
Priyambada, S., Er, M., Yahya, B. and Usagawa, T. (2021). Profile-based cluster evolution analysis: Identification of migration patterns for understanding student learning behavior. IEEE Access, 9(n/a), 101718–2.
Qi, C. and Liu, S. (2021). Evaluating on-line courses via reviews mining. IEEE Access, 9(n/a), 35451–39.
Qu, Y., Li, F., Li, L., Dou, X. and Wang, H. (2022). Can we predict student performance based on tabular and textual data?. IEEE Access, 10(n/a), 86008–19.
Rahman, M., Watanobe, Y., Matsumoto, T., Kiran, R. and Nakamura, K. (2022). Educational data mining to support programming learning using problem-solving data. IEEE Access, 10(n/a), 26186–202.
Shao, Z., Sun, H., Wang, X. and Sun, Z. (2020). An optimized mining algorithm for analyzing students’ learning degree based on dynamic data. IEEE Access, 8(n/a), 113543–56.
Siddaway, A. (2014). What is a systematic literature review and how do I do one. University of Stirling, 1(1), 1–13.
Siemens, G. and Baker, R.S. (2012). Learning analytics and educational data mining: towards communication and collaboration. In: Proceedings of the 2nd International Conference on Learning Analytics and Knowledge, ACM, Vancouver, British Columbia, Canada, 04/2012.
Wen, Y., Tian, Y., Wen, B., Zhou, Q., Cai, G. and Liu, S. (2020). Consideration of the local correlation of learning behaviors to predict dropouts from MOOCs. Tsinghua Science and Technology, 25(3), 336–47.
Xiao, X., Sun, R., Yao, Z., Zhang, C. and Chen, X. (2021). A Novel framework with weighted heterogeneous educational network embedding for personalized freshmen recommendation under the impact of COVID-19 storm. IEEE Access, 9(n/a), 67129–42.
Zeng, Y., Ouyang, Y., Gao, R., Qiu, Y., Yu, Y. and Wang, C. (2020). HHA: An attentive prediction model for academic abnormality. IEEE Access, 8(n/a), 124755–66.
Zhai, G., Yang, Y., Wang, H. and Du, S. (2020). Multi-attention fusion modeling for sentiment analysis of educational big data. Big Data Mining and Analytics, 4(3), 311–9.