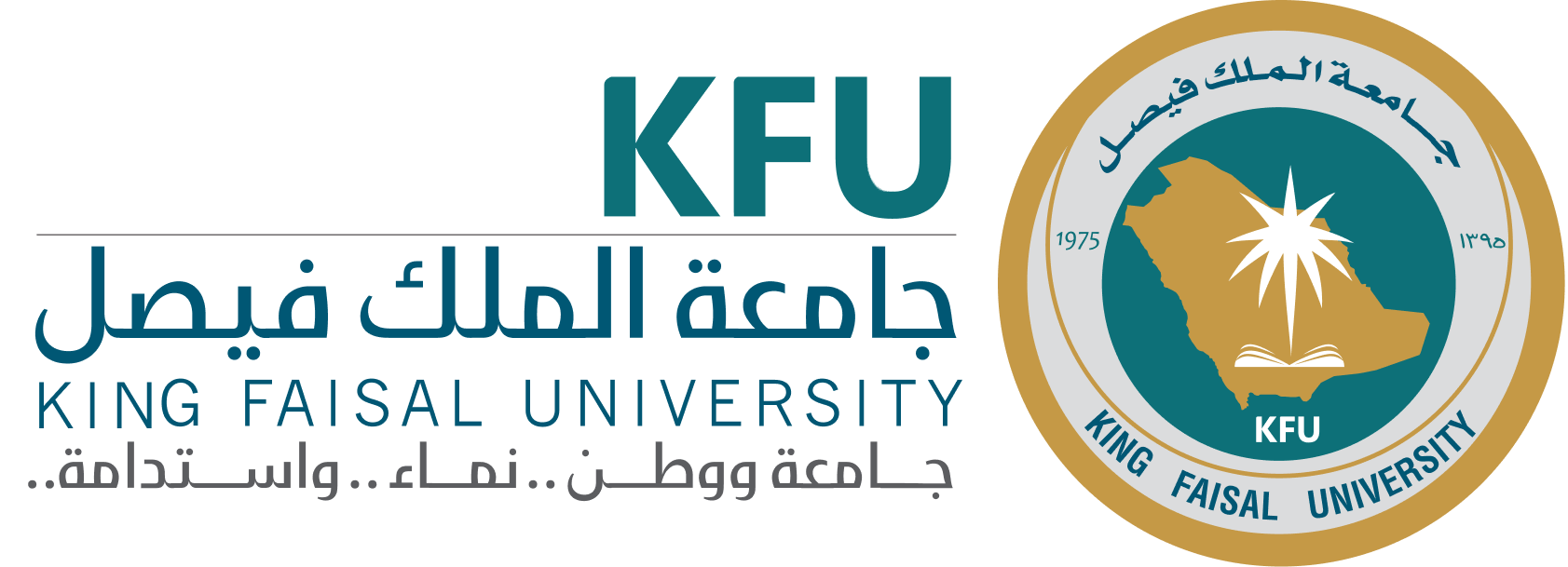
Scientific Journal Of King Faisal University
Basic and Applied Sciences
Scientific Journal of King Faisal University / Basic and Applied Sciences
Predicting Shear Stress Parameters in Consolidated Drained Conditions Using Artificial Intelligence Methods
(Benbouras Mohammed Amin)Abstract
Using the direct shear stress test for estimating shear stress parameters is considered to be of great importance, mainly for enhancing and strengthening soils, assessing their bearing capacity, and predicting potential risks that could bring harm to foundations. However, conducting the test in consolidated drained conditions is quite expensive and time-consuming (e.g., up to three months in consolidated clay). To our knowledge, few researchers have suggested simple models in undrained conditions to experimentally estimate these parameters. However, in large projects and slope studies, testing in consolidated drained conditions is more important because these conditions mimic reality. The current study aims to suggest a new model for estimating shear stress parameters. The reliability of the approach was tested through comparing several models of multiple regression analysis, genetic programming, and artificial neural networks. These models were tested on 98 samples of Algiers soil. The results showed the efficiency of the artificial neural network method with two hidden layers, which provided the best appropriate model, and the most approached results to experimental data, as compared with the other models. Based on these findings, this study proposes a structural flowchart for effectively predicting shear stress parameters effectively in future studies.
KEYWORDS
Artificial neural networks, cross-validation approach, direct shear test, genetic programming, multiple regression analysis, shear stress parameters
PDF
References
Alavi, A.H., Gandomi, A.H., Mollahasani, A. and Bazaz, J.B. (2013). 'Linear and tree-Based genetic programming for solving geotechnical engineering problems'. In: X. S. Yang, A. H. Gandomi, S. Talatahari, and A. H. Alavi, (eds.) Metaheuristics in Water, Geotechnical and Transport Engineering. Newnes, Australia: Elsevier. Doi: 10.1016/B978-0-12-398296-4.00012-X
Baykasoglu, A., Gullu, H., Canakci, H. and Ozbakir, L. (2008). Prediction of compressive and tensile strength of limestone via genetic programming. Expert Systems with Applications, 35(2), 111–23. Doi: 10.1016/j.eswa.2007.06.006
Benbouras, M.A. and Petrisor, A.I. (2021). Prediction of swelling index using advanced machine learning techniques for cohesive soils. Applied Sciences, 11(2), 5–36. Doi: 10.3390/app11020536.
Benbouras, M.A., Kettab Mitiche, R., Zedira, H., Petrisor, A.I., Mezouar, N. and Debiche, F. (2019). A new approach to predict the compression index using artificial intelligence methods. Marine Georesources and Geotechnology, 37(6), 704–20. DOI: 10.1080/1064119X.2018.1484533
Benbouras, M.A., Kettab, R.M., Debiche, F., Lagaguine, M., Mechaala, A., Bourezak, C. and Petrişor, A.-I. (2018a). Use of geotechnical and geographical information systems to analyze seismic risk in Algiers area. Revista Şcolii Doctorale de Urbanism, 3(1), 15–24.
Benbouras, M.A., Kettab, R.M., Zedira, H., Debiche, F. and Zaidi, N. (2018b). Comparing nonlinear regression analysis and artificial neural networks to predict geotechnical parameters from standard penetration test. Urbanism Architecture Constructions, 9(1), 275–88.
Benbouras, M.A., Kettab, R.M., Zedira, H., Petrisor, A.-I. and Debiche, F. (2017). Dry density in relation to other geotechnical proprieties of Algiers clay. Revista Şcolii Doctorale d’Urbanism, 2(1), 5–14.
Bowles, L.E. (1996). Foundation Analysis and Design. New York, United States: McGraw-hill.
Breiman, L. and Spector, P. (1992). Submodel selection and evaluation in regression, the X-Random Case. International Statistical Review, 60(3), 291–319. DOI: 10.2307/1403680
Çanakcı, H., Baykasoğlu, A. and Güllü, H. (2009). Prediction of compressive and tensile strength of Gaziantep basalts via neural networks and gene expression programming. Neural Computing and Applications, 18(8), 1031–41. Doi: 10.1007/s00521-008-0208-0
Collotta, T., Cantoni, R., Pavesi, U., Ruberl, E. and Moretti, P.C. (1989). A correlation between residual friction angle, gradation and the index properties of cohesive soils. Geotechnique, 39(2), 33–45. Doi: 10.1680/geot.1989.39.2.343
Das, S.K. and Basudhar, P. K. (2008). Prediction of residual friction angle of clays using artificial neural network. Engineering Geology, 100(3), 142–5. Doi: 10.1016/j.enggeo.2008.03.001
Debiche, F., Kettab, R.M., Benbouras, M.A., Benbellil, B., Djerbal, L. and Petrisor, A.-I. (2018). Use of GIS systems to analyze soil compressibility, swelling and bearing capacity under superficial foundations in Algiers region, Algeria. Urbanism Architecture Constructions, 9(2), 357–70.
Goetz, J.N., Brenning, A., Petschko, H. and Leopold, P. (2015). Evaluating machine learning and statistical prediction techniques for landslide susceptibility modeling. Computers and Geosciences, 81(1), 1–11. Doi: 10.1016/j.cageo.2015.04.007
Hatanaka, M. and Uchida, A. (1996). Empirical correlation between penetration resistance and internal friction angle of sandy soils. Soils and Foundations, 36(4), 1–9. DOI: 10.3208/sandf.36.4_1
Hunt, R. (2006). Geotechnical Investigation Methods: A Field Guide for Geotechnical Engineers. New York, United States: CRC Press. DOI: 10.1201/9781420042757
Kayadelen, C., Günaydın, O., Fener, M., Demir, A. and Özvan, A. (2009). Modeling of the angle of shearing resistance of soils using soft computing systems. Expert Systems with Applications, 36(9), 11814–26. DOI: 10.1016/j.eswa.2009.04.008
Khanlari, G.R., Heidari, M., Momeni, A.A. and Abdilor, Y. (2012). Prediction of shear strength parameters of soils using artificial neural networks and multivariate regression methods. Engineering Geology, 131(1), 11–8. DOI:10.1016/j.enggeo.2011.12.006
Lee, S.J., Lee, S.R. and Kim, Y.S. (2003). An approach to estimate unsaturated shear strength using artificial neural network and hyperbolic formulation. Computers and Geotechnics, 30(6), 489–503. DOI:10.1016/S0266-352X(03)00058-2
Mesri, G. and Shahien, M. (2003). Residual shear strength mobilized in first-time slope failures. Journal of Geotechnical and Geoenvironmental Engineering, 129(1), 12–31. DOI: 10.1061/(ASCE)1090-0241(2003)129:1(12)
Onyejekwe, S., Kang, X. and Ge, L. (2015). Assessment of empirical equations for the compression index of fine-grained soils in Missouri. Bulletin of Engineering Geology and the Environment, 74(3), 705–16. DOI: 10.1007/s10064-014-0659-8
Shahin, M.A. (2013). 'Artificial intelligence in geotechnical engineering: Applications, modeling aspects, and future directions'. In: X. S. Yang, A. H. Gandomi, S. Talatahari, and A. H. Alavi, (eds.) Metaheuristics in Water, Geotechnical and Transport Engineering. Newnes, Australia: Elsevier.
Shahin, M.A., Jaksa, M.B. and Maier, H.R. (2009). Recent advances and future challenges for artificial neural systems in geotechnical engineering applications. Advances in Artificial Neural Systems, 9(1), 2–10. DOI: 10.1155/2009/308239.
Skempton, A.W. (1964). Long-term stability of clay slopes. Geotechnique, 14(2), 77–102.
Semahi, S., Benbouras, M.A., Mahar, W.A., Zemmouri, N. and Attia, S. (2020). Development of spatial distribution maps for energy demand and thermal comfort estimation in Algeria. Sustainability, 12(15), 60–66. DOI: 10.3390/su12156066.
Stark, T.D. and Eid, H.T. (1994). Drained residual strength of cohesive soils. Journal of Geotechnical Engineering, 120(5), 856–71. DOI: 10.1061/(ASCE)0733-9410(1994)120:5(856)
Tiryaki, B. (2008). Predicting intact rock strength for mechanical excavation using multivariate statistics, artificial neural networks, and regression trees. Engineering Geology, 99(2), 51–60.
Wesley, L.D. (2003). Residual strength of clays and correlations using Atterberg limits. Geotechnique, 53(7), 669–72. DOI: 10.1680/geot.2003.53.7.669
Wesley, L.D. (2004). Discussion-Residual strength of clays and correlation using Atterberg limits. Geotechnique, 54(7), 503–4. DOI: 10.1680/geot.2004.54.7.503