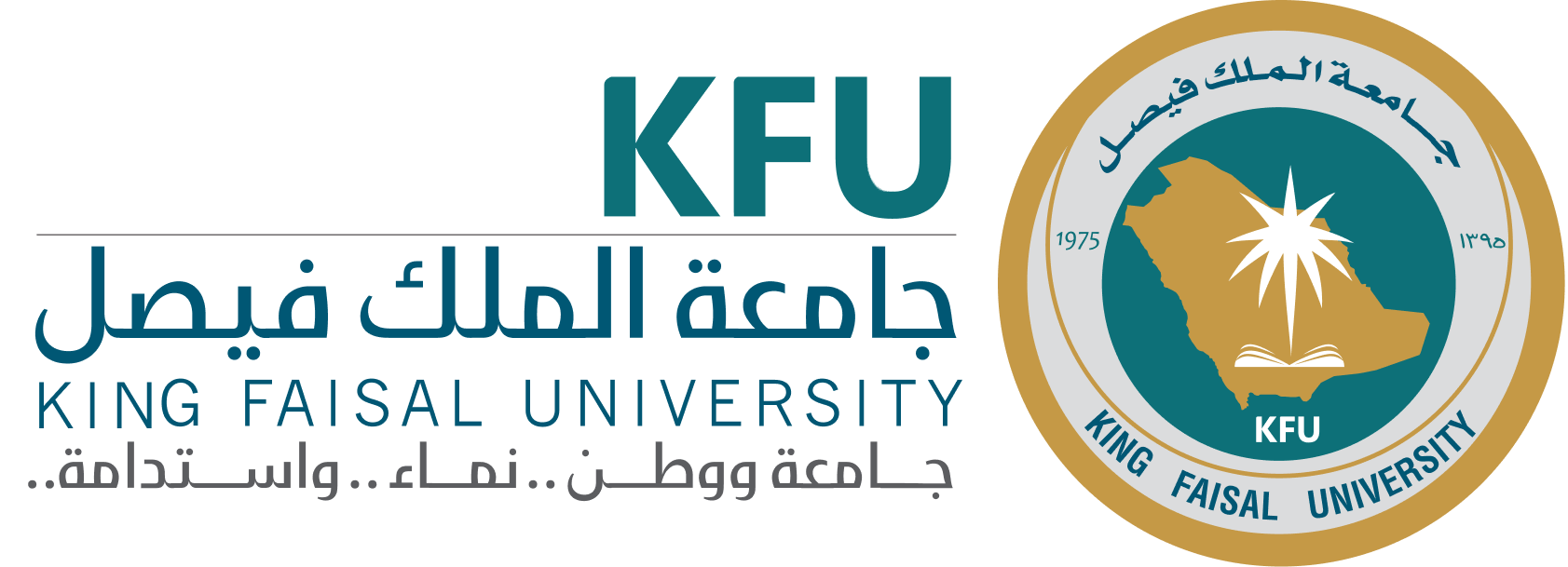
Scientific Journal Of King Faisal University: Basic and Applied Sciences
Scientific Journal of King Faisal University: Basic and Applied Sciences
Evaluating the Performance of Mixed Zero-Inflated Poisson Regression Models with Time-dependent and Time-independent Covariates
(Gadir Alomair)Abstract
One of the issues that researchers may encounter in count data is having many zeros. One of the solutions to model these data is using zero-inflated Poisson (ZIP) regression models. Recently, researchers have started to model longitudinal count data with time-dependent covariates. However, it has not been considered whether a model with time-dependent covariates provides a better fit than a model with time-independent covariates. In this paper, the fit between a mixed ZIP model with time-dependent covariates and a mixed ZIP model with time-independent covariates is compared using simulation. Using the deviance information criterion as a measure of fit, we found that the model with time-dependent covariates exhibits a better fit than the model with time-independent covariates.
KEYWORDS
correlated data, count data, excess zeros, longitudinal, mixed models, model fit
PDF
References
Agresti, A. (2006). An Introduction to Categorical Data Analysis. 2nd Edition. New York, USA: John Wiley and Sons, Inc. DOI: 10.1002/0470114754
Azen, R. and Walker, C. M. (2021). Categorical Data Analysis for the Behavioral and Social Sciences. 2nd Edition. USA: Routledge.
Ballinger, G.A. (2004). Using generalized estimating equations for longitudinal data analysis. Organizational research methods, 7(2), 127–150. DOI: 10.1177/1094428104263672
Baghfalaki, T. and Ganjali, M. (2020). A transition model for analysis of zero-inflated longitudinal count data using generalized poisson regression model. REVSTAT-Statistical Journal, 18(1), 27–45. DOI: 10.57805/revstat.v18i1.288
Baghfalaki, T. and Ganjali, M. (2021). Approximate Bayesian inference for joint linear and partially linear modeling of longitudinal zero-inflated count and time to event data. Statistical Methods in Medical Research, 30(6), 1484–1501. DOI: 10.1177/09622802211002868
Berg, A., Meyer, R. and Yu, J. (2004). Deviance information criterion for comparing stochastic volatility models. Journal of Business & Economic Statistics, 22(1), 107–120. DOI: 10.1198/073500103288619430
Burger, D.A., Schall, R., Jacobs, R. and Chen, D.G. (2019). A generalized Bayesian nonlinear mixed‐effects regression model for zero‐inflated longitudinal count data in tuberculosis trials. Pharmaceutical Statistics, 18(4), 420–432. DOI: 10.1002/pst.1933
Cameron, A.C. and Trivedi, P.K. (2013). Regression Analysis of Count Data . England: Cambridge University press.
Chen, E.Z. and Li, H. (2016). A two-part mixed-effects model for analyzing longitudinal microbiome compositional data. Bioinformatics, 32(17), 2611–2617. DOI: 10.1093/bioinformatics/btw308
Gibbons, R.D. and Hedeker, D. (2006). Longitudinal Data Analysis . New York, USA: John Wiley and Sons, Inc.
Hagen, T., Reinfeld, N. and Saki, S. (2023). Modeling of Parking Violations Using Zero-Inflated Negative Binomial Regression: A Case Study for Berlin. Transportation Research Record, 2677(6), 498–512.
Hagen, T., Reinfeld, N. and Saki, S. (2023). Modeling of Parking Violations Using Zero-Inflated Negative Binomial Regression: A Case Study for Berlin. Transportation Research Record, 2677(6), 498–512. DOI: 10.1177/03611981221148703
Hall, D.B. (2000). Zero-Inflated Poisson and Binomial Regression with Random Effects: A Case Study. Biometrics, 56(4), 1030–1039. DOI: 10.1111/j.0006-341x.2000.01030.x
Kamalja, K.K. and Wagh, Y.S. (2018). Estimation in zero-inflated Generalized Poisson distribution. Journal of Data Science, 16(1), 183–206. DOI: 10.6339/jds.201801_16 (1).0010
Lachenbruch, P.A. (2002). Analysis of data with excess zeros. Statistical Methods in Medical Research, 11(4), 297–302. DOI: 10.1191/0962280202sm289ra
Lalonde, T.L., Nguyen, A.Q., Yin, J., Irimata, K. and Wilson, J.R. (2013). Modeling correlated binary outcomes with time-dependent covariates. Journal of Data Science, 11(4), 715–738. DOI: 10.6339/jds.2013.11(4).1195
Lalonde, T.L. (2014). Modeling Longitudinal Count Data with Excess Zeros and Time-Dependent Covariates: Application to Drug Use. In: American Public Health Association (APHA) 142nd Annual Meeting and Exposition, New Orleans University, New Orleans, USA. 15-19/11/2014.
Lambert, D. (1992). Zero-inflated Poisson regression, with an application to defects in manufacturing. Technometrics, 34(1), 1–14. DOI: 10.2307/1269547
Liu, H. (2007). Growth curve models for zero-inflated count data: An application to smoking behavior. Structural Equation Modeling: A Multidisciplinary Journal, 14(2), 247–279. DOI: 10.1080/10705510709336746
McCullagh, P. (2019). Generalized Linear Models. Routledge. USA: Routledge.
Mekonnen, F.H., Lakew, W.D., Tesfaye, Z.D. and Swain, P.K. (2019). Statistical models for longitudinal zero-inflated count data: application to seizure attacks. African Health Sciences, 19(3), 2555–2564. DOI: 10.4314/ahs.v19i3.31
Miller, J.M. (2007). Comparing Poisson, Hurdle, and ZIP Model Fit Under Varying Degrees of Skew and Zero-inflation. PhD Thesis, University of Florida, Florida, USA.
Min, Y. and Agresti, A. (2005). Random effect models for repeated measures of zero-inflated count data. Statistical modelling, 5(1), 1–19. DOI: 10.1191/1471082x05st084oa
Misaii, H., Fouladirad, M. and Haghighi, F. (2024). Optimal task-driven time-dependent covariate-based maintenance policy. Journal of Computational and Applied Mathematics, 435(n/a), 115315. DOI: 10.1016/j.cam.2023.115315
Motalebi, N., Owlia, M.S., Amiri, A. and Fallahnezhad, M.S. (2023). Monitoring social networks based on Zero-inflated Poisson regression model. Communications in Statistics-Theory and Methods, 52(7), 2099–2115. DOI: 10.1080/03610926.2021.1945103
Neelon, B.H., O’Malley, A.J. and Normand, S.L.T. (2010). A Bayesian model for repeated measures zero-inflated count data with application to outpatient psychiatric service use. Statistical modelling, 10(4), 421–439. DOI: 10.1177/1471082x0901000404
Perumean-Chaney, S.E., Morgan, C., McDowall, D. and Aban, I. (2013). Zero-inflated and overdispersed: what’s one to do? Journal of Statistical Computation and Simulation, 83(9), 1671–1683. DOI: 10.1080/00949655.2012.668550
Pittman, B., Buta, E., Garrison, K. and Gueorguieva, R. (2023). Models for zero-inflated and overdispersed correlated count data: an application to cigarette use. Nicotine and Tobacco Research, 25(5), 996–1003. DOI: 10.1093/ntr/ntac253
Pooley, C.M. and Marion, G. (2018). Bayesian model evidence as a practical alternative to deviance information criterion. Royal Society Open Science, 5(3), 171519. DOI: 10.1098/rsos.171519
Spiegelhalter, D.J., Best, N.G., Carlin, B.P. and Van Der Linde, A. (2002). Bayesian measures of model complexity and fit. Journal of the Royal Statistical Society Series B: Statistical Methodology, 64(4), 583–639. DOI: 10.1111/1467-9868.00353
Tang, W., He, H. and Tu, X.M. (2023). Applied Categorical and Count Data Analysis. 2nd Edition. London, England: CRC Press.
Weiler, H. (1964). A significance test for simultaneous quanta1 and quantitative responses. Technometrics, 6(3), 273–285. DOI: 10.2307/1266044
Xia, Y., Sun, J., Chen, D.G., Xia, Y., Sun, J. and Chen, D.G. (2018). Modeling zero-inflated microbiome data. In. Y. Xia, J. Sun, D. Chen, Y. Xia, J. Sun and D. Chen (eds.) Statistical analysis of microbiome data with R. Singapore: Springer, ICSA Book Series in Statistics. DOI: 10.1007/978-981-13-1534-3_12
Zeger, S.L. and Liang, K.Y. (1992). An overview of methods for the analysis of longitudinal data. Statistics in medicine, 11(14‐15), 1825–1839. DOI: 10.1002/sim.4780111406
Zhang, Q. and Yi, G.Y. (2023). Zero‐inflated Poisson models with measurement error in the response. Biometrics, 79(2), 1089–1102. DOI: 10.1111/biom.13657
Zorn, C.J. (1996). Evaluating zero-inflated and hurdle Poisson specifications. Midwest Political Science Association, 18(20), 1–16.