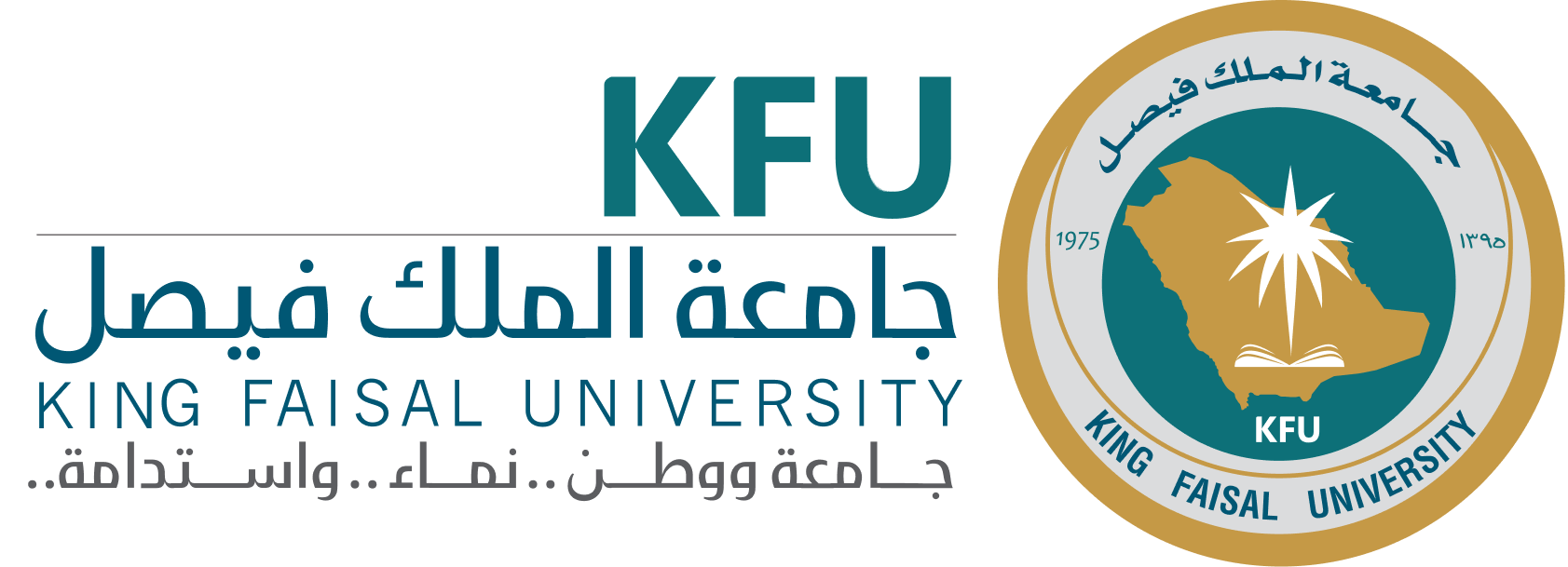
Scientific Journal Of King Faisal University: Basic and Applied Sciences
Scientific Journal of King Faisal University: Basic and Applied Science
Detecting Hadith Authenticity Using a Deep-learning Approach
(Eshrag Ali Refaee)Abstract
Hadith is a collection of texts containing sayings of the prophet Muhammad, which, along with accounts of his daily practice, constitute the second major source of legislation for Muslims after the Holy Koran. The Hadith collection comprises thousands of text pieces transferred over the years by many narrators with varying degrees of credibility. Hadith scholars are faced with the challenge of assessing the degree of a specific Hadith’s authenticity to classify the Hadith as Sahih (fully authentic and accepted) or Daif (rejected). Automatic Hadith classification has been addressed in the literature; however, the results vary and are not directly comparable, as no dataset has been made available for benchmarking. In addition, no previous work has utilised deep-learning (DL) approaches for Hadith classification. This work contributes by 1) collecting and publicly releasing a benchmark Hadith dataset of almost 4,000 Hadith texts to facilitate future research, 2) exploring DL model performance on binary Hadith classification tasks, and 3) benchmarking traditional machine learning against DL models. Our best results were recorded with an ARBERT DL model that provided an accuracy score of 91.56%.
KEYWORDS
Hadith classification; deep learning; Classical Arabic; machine learning; Hadith science; Hadith authenticity
PDF
References
Abainia, K. and Rebbani, H. (2019). Comparing the effectiveness of the improved ARLstem algorithm with existing Arabic light stemmers. In: Proceedings of the 2019 International Conference on Theoretical and Applicative Aspects of Computer Science (ICTAACS), (pp. 1–8), Skikda, Algeria, 15–6/12/2019.
Abdelaal, H.M., Ahmed, A.M., Ghribi, W. and Alansary, H.A.Y. (2019a). Knowledge discovery in the Hadith according to the reliability and memory of the reporters using machine learning techniques. IEEE Access, 7(n/a), 157741–55.
Abdelaal, H.M. and Youness, H.A. (2019b). Hadith classification using machine learning techniques according to its reliability. Science and Technology, 22(3), 259–71.
Duderija, A. (2009). Evolution in the canonical Sunni Hadith body of literature and the concept of an authentic Hadith during the formative period of Islamic ought as based on recent Western scholarship. Arab Law Quarterly, 23(4), 389–415.
Al Ma´ani Dictionary. (n/a). In Al Ma´ani Dictionary. Available at: https://www.almaany.com/en/ dict/ar-en/hadith/ (Accessed on 10/10/2021).
Aldhaln, K., Zeki, A., Zeki, A. and Alreshidi, H. (2012a). Improving knowledge extraction of Hadith classifier using decision tree algorithm. In: Proceedings of the 2012 International Conference on Information Retrieval and Knowledge Management. (pp. 148–152), Kuala Lumpur, Malaysia, 13–5/03/2012.
Aldhlan, K.A., Zeki, A.M., Zeki, A.M. and Alreshidi, H.A. (2012b). Novel mechanism to improve Hadith classifier performance. In: Proceedings of the 2012 International Conference on Advanced Computer Science Applications and Technologies (ACSAT). (pp. 512–517), Kuala Lumpur, Malaysia, 13-15/03/2012.
Antoun, W., Baly, F. and Hajj, H. (2020). AraBERT: Transformer-based model for Arabic language understanding. In: Proceedings of LREC 2020 Workshop Language Resources and Evaluation Conference. (p. 9), Marseille, France, 13–5/05/2020.
Avati, A., Jung, K., Harman, S., Downing, L. and Ng, A. (2018). Improving palliative care with deep learning. BMC Medical Informatics and Cecision Making, 18(4), 55–64.
Azmi, A.M., Al-Qabbany, A.O. and Hussain, A. (2019). Computational and natural language processing-based studies of Hadith literature: A survey. Artificial Intelligence Review, 52(2), 1369–414.
Baru, R., Omar, S.H.S., Ag, I.M., Fuad, A.N. and Mohd, M.F. (2017). Consolidation of Ulum Al-hadith to the society. International Journal of Academic Research in Business and Social Sciences, 7(10), 2222–6990.
Desilver, D. and Masci, D. (n/a). World’s Muslim Population, Pew Research Centre. Available at: https: //www.pewresearch.org/fact-tank/2017/01/31/worlds-muslim-population-more-widespread-than-you-might-think/ (Accessed on 12/11/2021).
El-Alami, F.Z., El Alaoui, S.O. and Nahnahi, N.E., (2021). Contextual semantic embeddings based on fine-tuned AraBERT model for Arabic text multi-class categorization. Journal of King Saud University-Computer and Information Sciences, In press.
European Languages Resources Association (ELRA). (n.d.). ELRA Universal Catalogue: ELRA–WC0134. Available at: http://universal.elra. info/product_info.php?cPath=42_43 and products_id=353/ (Accessed on 03/11/2021).
Fadele, A.A., Kamsin, A., Ahmad, K. and Hamid, H. (2021). A novel classification to categorise original Hadith detection techniques. International Journal of Information Technology, n/a(n/a), 1–15. DOI: https://doi.org/10.1007/s41870-021-00649-3
Ghazizadeh, M., Zahedi, M.H., Kahani, M. and Bidgoli, B.M. (2008). Fuzzy expert system in determining Hadith validity. In: Advances in Computer and Information Sciences and Engineering (pp. 354–359). Bridgeport, CT, USA. 03–12/12/2007.
Habash, N. (2010). Introduction to Arabic Natural Language Processing. United States: Synthesis Lectures on Human Language Technologies, Morgan and Claypool Publishers.
Johnson, J.M. and Khoshgoftaar, T.M. (2019). Survey on deep learning with class imbalance. Journal of Big Data, 6(1), 1–54.
Najeeb, M.M. (2014). Towards innovative system for Hadith isnad processing. International Journal of Computer Trends and Technology, 18(6), 257–59.
Najiyah, I., Susanti, S., Riana, D. and Wahyudi, M. (2017). Hadith degree classification for Shahih Hadith identification web based. In: Proceedings of the 2017 5th International Conference on Cyber and IT Service Management (CITSM) (pp. 1–6), Denpasar, Indonesia, 08–10/08/2017.
Patwa, P., Aguilar, G., Kar, S., Pandey, S. and PYKL, S. (2020). Semeval-2020 task 9: Overview of sentiment analysis of code-mixed tweets. In: Proceedings of the Fourteenth Workshop on Semantic Evaluation. (pp. 774–790). Barcelona, Spain, 12–3/12/2020.
Refaee, E. (2017). Sentiment analysis for micro-blogging platforms in Arabic. In: Proceedings International Conference on Social Computing and Social Media (pp. 275–294). Proceedings: Cham, Springer, Vancouver, Canada, 15–20/07/2017.
UNESCO. (n.d.). UNESCO: World Arabic Language Day. Available at: https://en.unesco.org/commemorations/worldarabiclanguageday (Accessed on 02/10/2021).
Zerrouki, T. (2010). PyArabic, an Arabic Language Library for Python. Available at: https://pypi.org/project/PyArabic/ (Accessed on 25/09/2021).