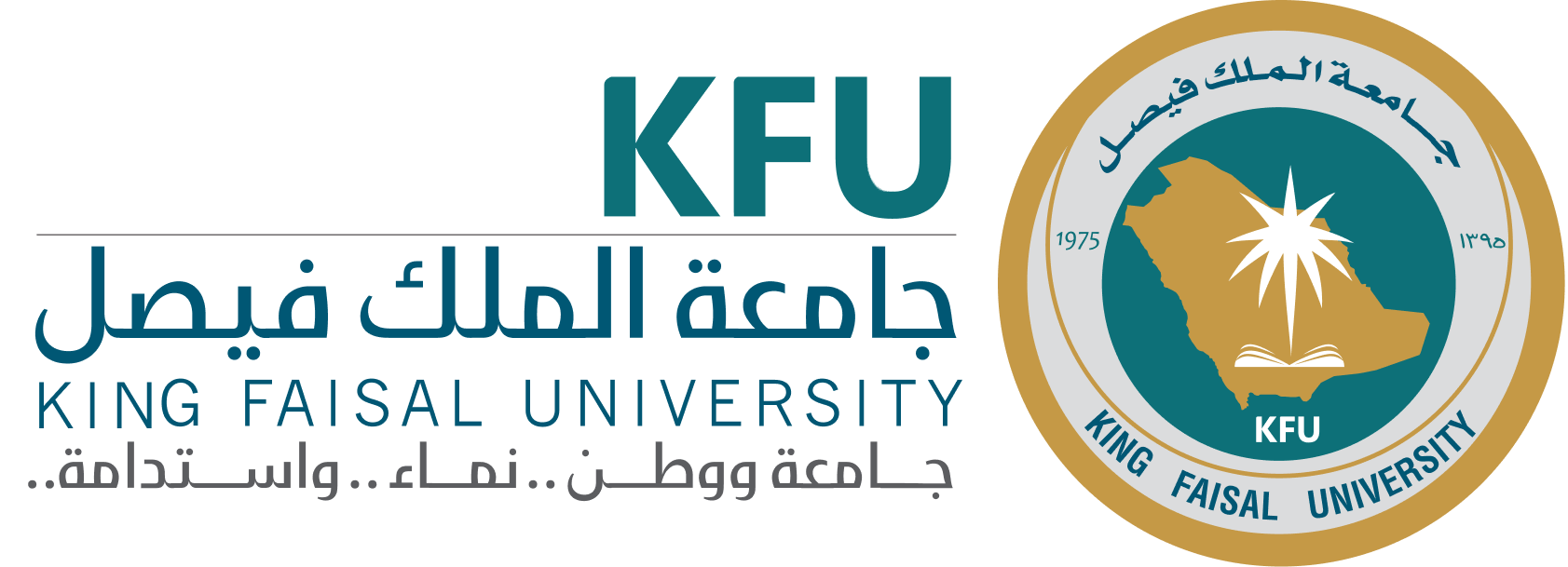
Scientific Journal Of King Faisal University
Basic and Applied Sciences
Scientific Journal of King Faisal University / Basic and Applied Sciences
Diagnosis of Diseases Based on Iridology Using Fuzzy Logic
(Zakaria Madhouse , Ammar Kayli and Luna Himmami)Abstract
Many automatic methods have been introduced in iridology to predict diseases according to the iridology chart. This is important to prevent diseases before they develop. This research aims to find a computer model for the early diagnosis of diseases in the brain, back, pelvis, abdomen, and chest using the iridology chart based on fuzzy logic. Image preprocessing for the iris aims to find the ring, code, and features of the iris. Five fuzzy models have been built for diagnosis and to determine a person's disease rate based on specific features that were extracted from the iris as the input variables. Each model contains four membership functions for each input or output variables and 64 fuzzy rules for fuzzification and defuzzification. The five models that were built to diagnose the five diseases of iridology have an accuracy rate of over 98%, with an average accuracy of 98.6223%. The results mean that the models are qualified for use by doctors as medical tools to diagnose specific diseases or as a tool for the public to reassure them about their health.
KEYWORDS
Abdominal, back, brain, chest, fuzzification, pelvic
PDF
References
Amerifar, S., Targhi. A.T. and Dehshibi., M.M. (2015). Iris the picture of health: towards medical diagnosis of diseases based on iris pattern. In: The Tenth International Conference on Digital Information Management (ICDIM), Jeju, South Korea, 21–23/10/2015, DOI: 10.1109/ICDIM.2015.7381861.
Andana, S.N., Novamizanti, L. and Ramatryana, A. (2019). Measurement of cholesterol conditions of eye image using fuzzy local binary pattern (flbp) and linear regression. In: IEEE International Conference on Signals and Systems (ICSigSys), Bandung, Indonesia, Indonesia, 16–18/07/2019.
Arvacheh, E. M. (2006). A Study of Segmentation and Normalization for Iris Recognition Systems. Master’s Dissertation, Waterloo University, Ontario, Canada.
Bansal, A., Agarwal, R. and Sharma, R.K. (2018). Iris images based pre-diagnostic tool to predict obstructive lung diseases, Biomedical Research, Special Section: Medical Diagnosis and Study of Biomedical Imaging Systems and Applications, n/a(Special Issue ), 517–22. DOI: 10.4066/biomedicalresearch.29-17-1998.
Dobes, M., Martinek, J., Skoupil, D., Dobešová, Z. and Pospíšil, J. (2006). Human eye localization using the modified hough transform. Elsevier Optik, 117(10), 468–73.
Dobes, M., Machala, L., Tichavský, P., and Pospíšil, J. (2004). Human Eye Iris Recognition Using the Mutual Information. Elsevier Optik, 115(9), 399–405.
Dobes, M. and Machala,L. (2004), UPOL Iris Image Database. Available at: http://phoenix.inf.upol.cz/iris/ (accessed on 10/10/2020).
Dubois, D. and Prade, H. (1992). Fuzzy rules in knowledge-based systems. In: R. Ronald, R. Yager, and L.A. Zadeh (eds.) An Introduction to Fuzzy Logic Applications in Intelligent Systems. Boston, MA: Springer.
Hassanzad, M., Orooji, A., Valinejadi, A. and Velayati, A. (2017). A fuzzy rule-based expert system for diagnosing cystic fibrosis. Electron Physician, 9(12), 5974–84, doi: 10.19082/5974.
Hernández, F., Vega, R., Tapia1, F., Morocho, D. and Fuertes, W. (2019). Early detection of Alzheimer's using digital image processing through iridology: An alternative method. Advances in Science, Technology and Engineering Systems Journal, 4(3), 126–37.
Inan. O., Arslan, D., Tasdemir, S. and Ozcan, M.M. (2011). Application of fuzzy expert system approach on prediction of some quality characteristics of grape juice concentrate (Pekmez) after different heat treatments.
J. Food Sci Technol, 48(4), 423–31.
Jensen, B. and Bodeen, D.V. (1991). Visions of Health: Understanding Iridiology. New York, NY: Penguin Publishing Group
Jogi, S.P. and Sharma, B.B. (2014). Methodology of Iris Image analysis for clinical diagnosis. In: International Conference on Medical Imaging, m-Health and Emerging Communication Systems (MedCom), Greater Noida, India, 7–8/11/2014. DOI: 10.1109/MedCom.2014.7006010.
Kusumaningtyas, E.M., Barakbah, A. and Hermawan, A.A. (2017). Feature extraction for application of heart abnormalities detection through iris based on mobile devices. EMITTER International Journal of Engineering Technology, 5(2), 312–27.
Lane, H.E. (2012). Diagnosis from the Eye, a New Art of Diagnosing with Perfect Certainty from the Iris of the Eye the Normal and Abnormal Conditions of the Organism in General and of the Different Organs in Particular. A Scientific Essay for the Public and Medical Professi, Rarebooksclub.com.
Narayanan, S.J., Paramasivam, I., Bhatt, R.B. and Khalid, M. (2015). A study on the approximation of clustered data to parameterized family of fuzzy membership functions for the induction of fuzzy decision trees. Cybernetics and Information Technologies, 15(2), 75–96, DOI: 10.1515/cait-2015-0030.
Nasrolahzadeh, M., Mohammadpoori, Z. and Haddadnia, J. (2016). Analysis of mean square error surface and its corresponding contour plots of spontaneous speech signals in Alzheimer's disease with adaptive wiener filter. Computers in Human Behavior, 61(n/a), 364–71, doi: 10.1016 j.chb.2016.03.031.
Novák, V., Perfilieva, I., and Močkoř, J. (1999). Mathematical Principles of Fuzzy Logic. Boston, MA: Springer.
Pujari, V., Surve, R., Thanekar, K. and Panchal, K. (2019). Iris analysis for health diagnosis. International Journal of Innovative Science and Research Technology, 4(4), 303–07.
Parashar, R. and Joshi, S. (2012). Comparative study of iris databases and UBIRIS database for iris recognition methods for non-cooperative environment. International Journal of Engineering Research & Technology (IJERT), 1(5), n/a.
Ray, A., Mahapatra, N., Das, S.S. and Mishra, A. (2018). Iris recognition using gabor filter and SURF feature detection technique. The IUP Journal of Information Technology, 14(2), 53–61.
Samant, P., and Agarwal, R. (2018). Machine learning techniques for medical diagnosis of diabetes using iris images. Computer Methods and Programs in Biomedicine, 157(n/a), 121–8. DOI: 10.1016/j.cmpb.
Sivasankar, K., Sujaritha, M., Pasupathi, P. and Muthukumar, S. (2012). FCM based iris image analysis for tissue imbalance stage identification. In: International Conference on Emerging Trends in Science, Engineering and Technology (INCOSET), Tiruchirappalli, India, 13–14/12/2012. DOI: 10.1109/INCOSET.2012.6513907.
Shankar, S.G. and Manikandan, K. (2019). Diagnosis of diabetes diseases using optimized fuzzy rule set by Grey Wolf optimization. Elsevier Pattern Recognition Letters, 125(n/a), 432–8. DOI: 10.1016/j.patrec.2019.06.005.
Wibawa, A.D, Sitorus, M. A. R. and Purnomo, M. H. (2016). Classification of iris image of patient chronic renal failure (CRF) using watershed algorithm and support vector machine (SVM). Journal of Theoretical and Applied Information Technology, 91(2),390–6.