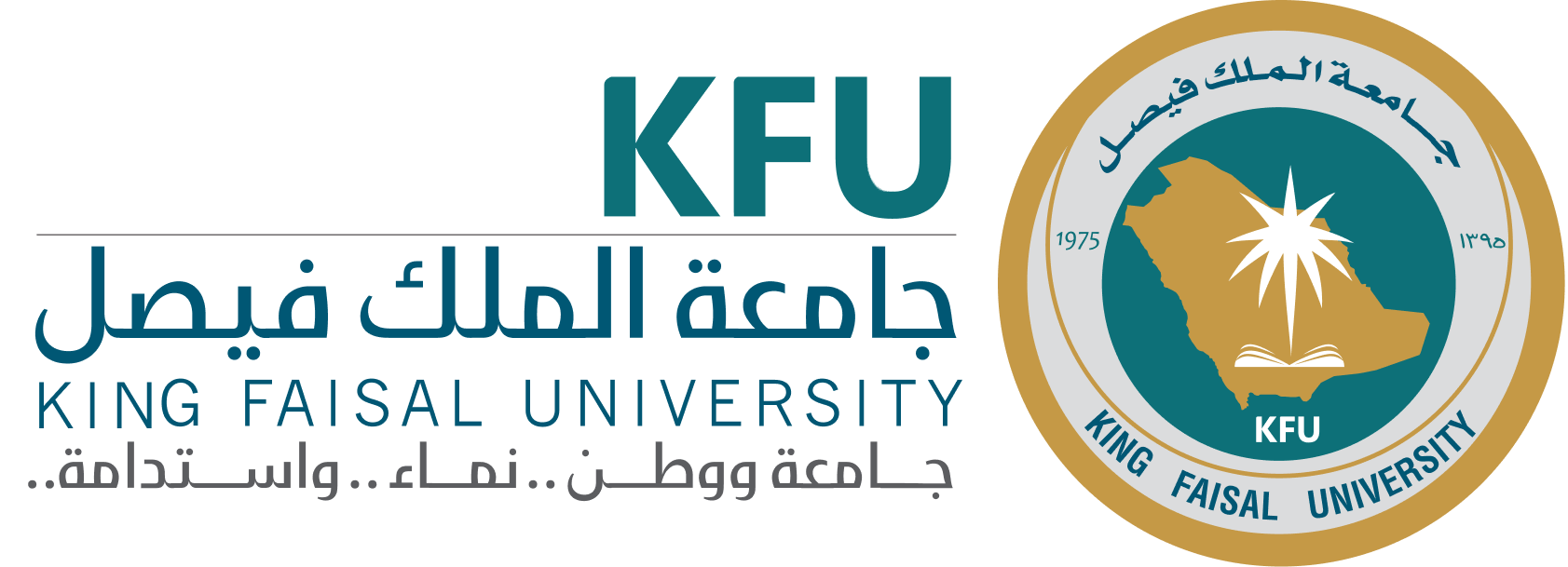
Scientific Journal Of King Faisal University: Basic and Applied Sciences
Scientific Journal of King Faisal University: Basic and Applied Sciences
Minimising the Heat Affected Zone in the Laser Cutting of Ti-6Al-4V Sheets using the Monte Carlo Method
(Ekhlas Jabir Mahmood)Abstract
This research attempts to minimise the heat affected zone (HAZ) width that forms in the laser cutting process of Ti-6Al-4V sheets. The experimental results of the HAZ for 32 sets of five CO2 laser cutting parameters (with the assist pressure of argon gas) were used to build the artificial neural network (ANN) model, each with a different material thickness, cutting speed, laser beam power, assist gas pressure and lens focal length percentage. A relationship formula was derived by connecting the laser cutting parameters based on the connection weights obtained from the developed ANN model. The MAPE value for the comparison between the predicted and experimental HAZ width was 4.192%. The Monte Carlo optimisation method was performed using 2,000 simulations to identify a suitable optimal solution. The results showed that the most effective transfer function type in the hidden layers was the linear function. The model was highly sensitive for the cutting speed (CS), assist gas pressure (GP) and beam power (BP) parameters and less sensitive for the parameters of thickness (T) and lens focal length (LFL). The optimum values of the laser cutting parameters that produced a minimum value of HAZ was T = 1 mm, LFL = 30%, BP = 3 kW, CS = 1 m/min and GP = 14 bars.
KEYWORDS
CO2 laser, artificial neural network (ANN), cutting speed, beam power, assist gas pressure
PDF
References
Akbari, M., Saedodin, S., Panjehpour, A., Hassani, M., Afrand, M. and Torkamany, M. J. (2016). Numerical simulation and designing artificial neural network for estimating melt pool geometry and temperature distribution in laser welding of Ti6Al4V alloy. Optik, 127(23), 11161–72.
Almeida, I. A., De Rossi, W., Lima, M. S. F., Berretta, J. R., Nogueira, G. E. C., Wetter, N. U. and Vieira Jr, N. D. (2006). Optimization of titanium cutting by factorial analysis of the pulsed Nd: YAG laser parameters. Journal of Materials Processing Technology, 179(1–3), 105–10.
Biswas, R., Kuar, A. S., Biswas, S. K. and Mitra, S. (2010). Artificial neural network modelling of Nd: YAG laser microdrilling on titanium nitride–alumina composite. Proceedings of the Institution of Mechanical Engineers, Part B: Journal of Engineering Manufacture, 224(3), 473–82.
Desai, C. K. and Shaikh, A. (2012). Prediction of depth of cut for single-pass laser micro-milling process using semi-analytical, ANN and GP approaches. The International Journal of Advanced Manufacturing Technology, 60(9–12), 865–82.
Cybenko, G. (1992). Approximation by superpositions of a sigmoidal function. Mathematics of Control, Signals and Systems, 5(4), 455.
Dahotre, N. B. and Harimkar, S. P. (2008). Laser Fabrication and Machining of Materials, Springer: Berlin.
Davim, J. P., Barricas, N., Conceicao, M. and Oliveira, C. (2008). Some experimental studies on CO2 laser cutting quality of polymeric materials. Journal of Materials Processing Technology, 198(1–3), 99–104.
Dhara, S. K., Kuar, A. S. and Mitra, S. (2008). An artificial neural network approach on parametric optimization of laser micro-machining of die-steel. The International Journal of Advanced Manufacturing Technology, 39(1–2), 39–46.
Dubey, A. K. and Yadava, V. (2008). Multi-objective optimisation of laser beam cutting process. Optics and Laser Technology, 40(3), 562–70.
Eltawahni, H. A., Olabi, A. G. and Benyounis, K. Y. (2011). Investigating the CO2 laser cutting parameters of MDF wood composite material. Optics and Laser Technology, 43(3), 648–59.
Feng, C. X. J., Yu, Z. G. and Kusiak, A. (2006). Selection and validation of predictive regression and neural network models based on designed experiments. IIE Transactions, 38(1), 13–23.
Garson, D. G. (1991). Interpreting neural network connection weights. AI Expert, 6(4), 47–51.
Ghany, K. A. and Newishy, M. (2005). Cutting of 1.2 mm thick austenitic stainless steel sheet using pulsed and CW Nd: YAG laser. Journal of Materials Processing Technology, 168(3), 438–47.
Hamoudi, W. K. (1997). The effects of speed and processing gas on laser cutting of steel using a 2 kW CO sub 2 laser. International Journal for the Joining of Materials (Denmark), 9(1), 31–6.
Hornik, K., Stinchcombe, M. and White, H. (1989). Multilayer feedforward networks are universal approximators. Neural Networks, 2(5), 359–66.
Kamrunnahar, M. and Urquidi-Macdonald, M. (2011). Prediction of corrosion behaviour of Alloy 22 using neural network as a data mining tool. Corrosion Science, 53(3), 961–7.
Kroese, D. P., Brereton, T., Taimre, T. and Botev, Z. I. (2014). Why the Monte Carlo method is so important today. Wiley Interdisciplinary Reviews: Computational Statistics, 6(6), 386–92.
Lee, H. T. and Te Chen, C. (2011). Numerical and experimental investigation into effect of temperature field on sensitization of AISI 304 in butt welds fabricated by gas tungsten arc welding. Materials Transactions, 52(7), 1506–14.
Liao, T. W. and Chen, L. J. (1994). A neural network approach for grinding processes: modelling and optimization. International Journal of Machine Tools and Manufacture, 34(7), 919–37.
Madić, M. J. and Radovanović, M. R. (2012). Analysis of the heat affected zone in CO2 laser cutting of stainless steel. Thermal Science, 16(suppl. 2), 363–73.
Mahmood, A. J. (2018). Theoretical study of comparative between the speed of penetration and cutting using a laser beam. Engineering and Technology Journal, 36(Part B Scientific), 37–43.
Miraoui, I., Boujelbene, M. and Bayraktar, E. (2013). Effects of laser cutting main parameters on microhardness and microstructure changes of stainless steel. In Advanced Materials Research, 664(n/a), 811–16.
Miraoui, I., Zaied, M. and Boujelbene, M. (2014). Effect of laser beam diameter on cut edge of steel plates obtained by laser machining. Applied Mechanics and Materials, 467(n/a), 227–32.
Nagarajan, R. (2000). Parametric Study of the Effect of Laser Cutting Variables on the Cut Quality. PhD Thesis, Wichita State University, Kansas, United States of America.
Pandey, A. and Dubey, A. (2012). Simultaneous optimization of multiple quality characteristics in laser cutting of titanium alloy sheet. Optics and Laser Technology, 44(6), 1858–65.
Quintero, F., Pou, J., Lusquinos, F., Boutinguiza, M., Soto, R. and Perez-Amor, M. (2004). Quantitative evaluation of the quality of the cuts performed on mullite-alumina by Nd: YAG laser. Optics and Lasers in Engineering, 42(3), 327–40.
Radovanovic, M. and Madic, M. (2011). Experimental investigations of CO2 laser cut quality: a review. Nonconventional Technologies Review, 15(4), 35–42.
Sarkar, S., Mitra, S. and Bhattacharyya, B. (2006). Parametric optimisation of wire electrical discharge machining of γ titanium aluminide alloy through an artificial neural network model. The International Journal of Advanced Manufacturing Technology, 27(5), 501–08.
Sheng, P. S. and Joshi, V. S. (1995). Analysis of heat-affected zone formation for laser cutting of stainless steel. Journal of Materials Processing Technology, 53(3–4), 879–92.
Shiue, R. K., Chang, C. T., Young, M. C. and Tsay, L. W. (2004). The effect of residual thermal stresses on the fatigue crack growth of laser-surface-annealed AISI 304 stainless steel: Part I: computer simulation. Materials Science and Engineering, 364(1), 101–8.
Topçu, İ. B., Boga A. R. and Hocaoglu F. O. (2009). Modeling corrosion currents of reinforced concrete using ANN. Automation in Construction, 18(2), 145–152.
Trochim, W. M., and Donnelly, J. P. (2001). Research Methods Knowledge Base. Cincinnati, OH: Atomic Dog Publishing.
Wei, Y., Xu, Y., Dong, Z. and Zhan, X. (2009). Three-dimensional Monte Carlo simulation of discontinuous grain growth in HAZ of stainless steel during GTAW process. Journal of Materials Processing Technology, 209(3), 1466–70.
Yusoff, N., Saifu, R. I., Azuddin, M. and Aznijar, A. (2008). Selected Malaysian wood CO2-laser cutting parameters and cut quality. American Journal of Applied Sciences, 5(8), 990–6.